OUR PARTNERS
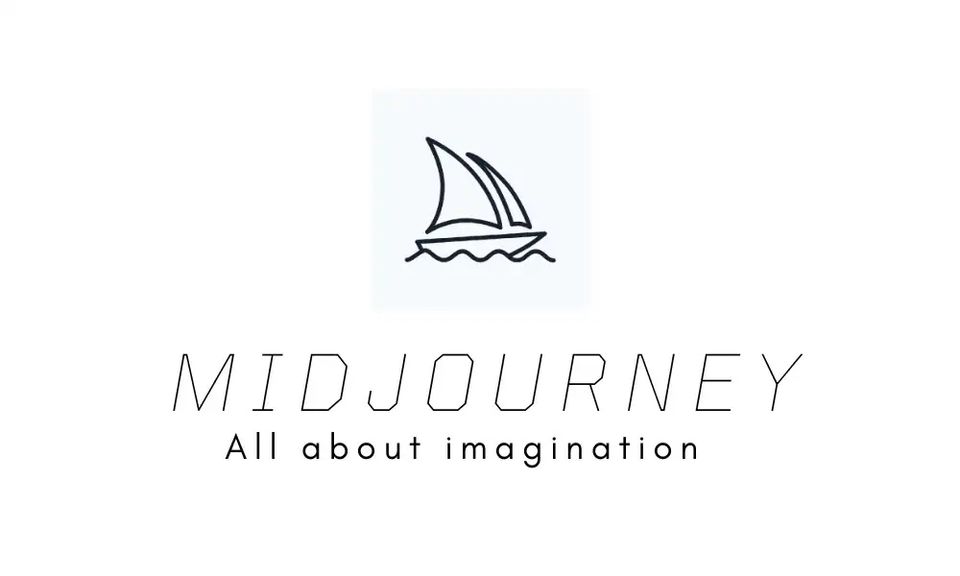
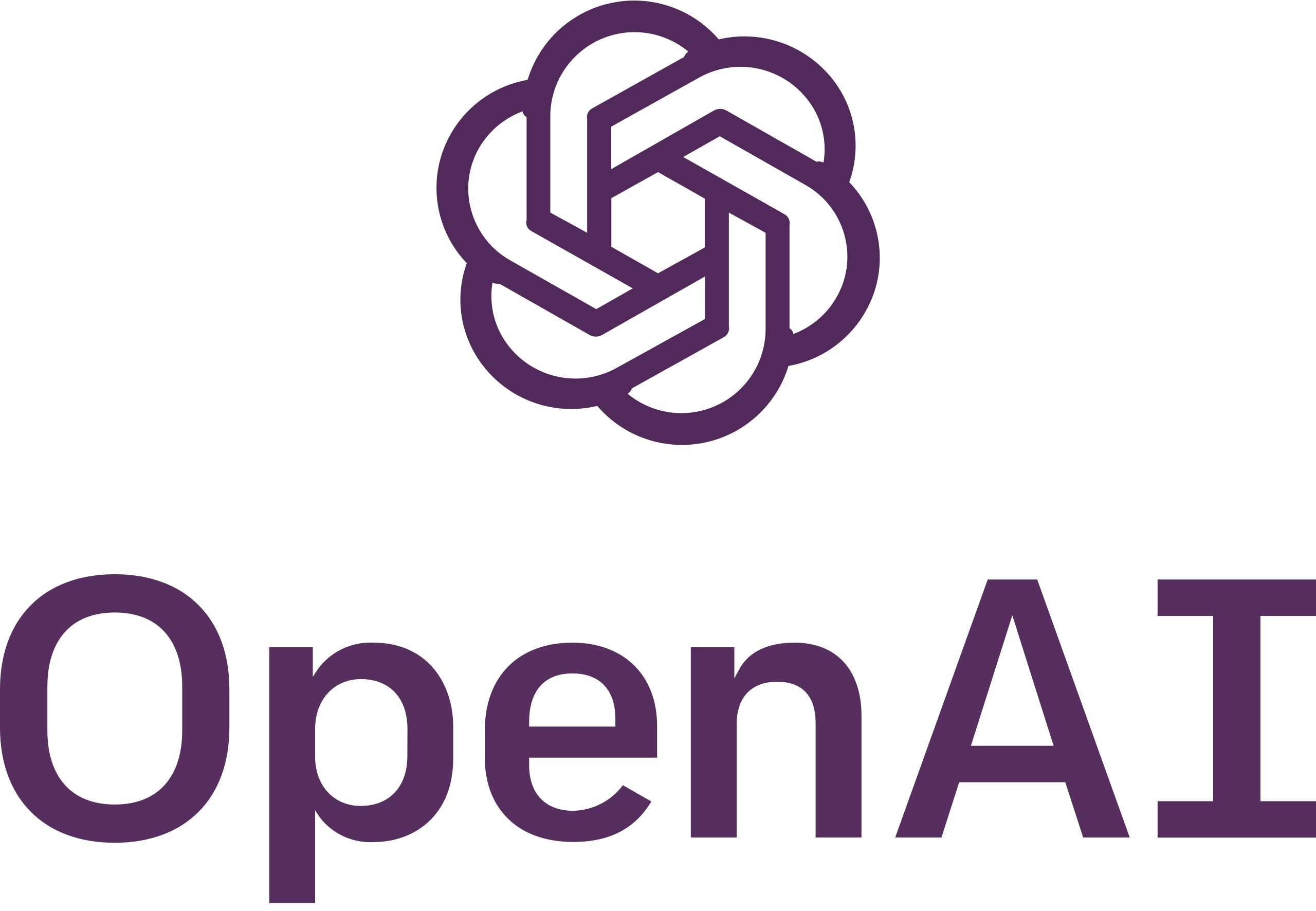
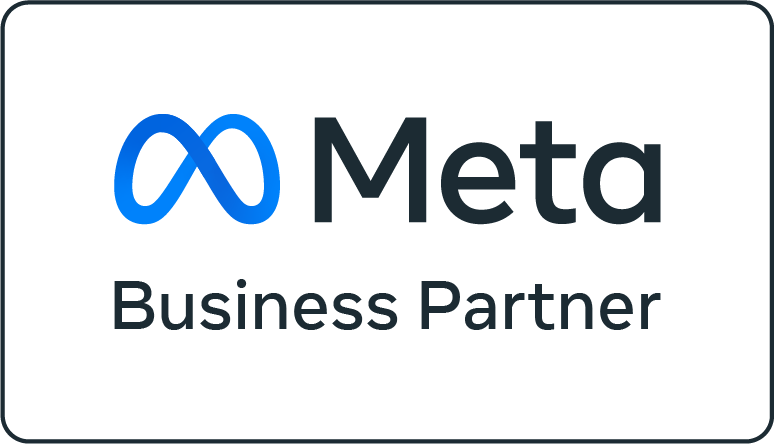
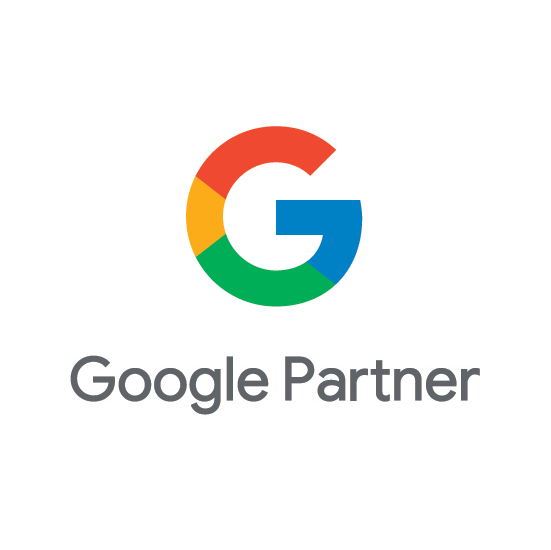
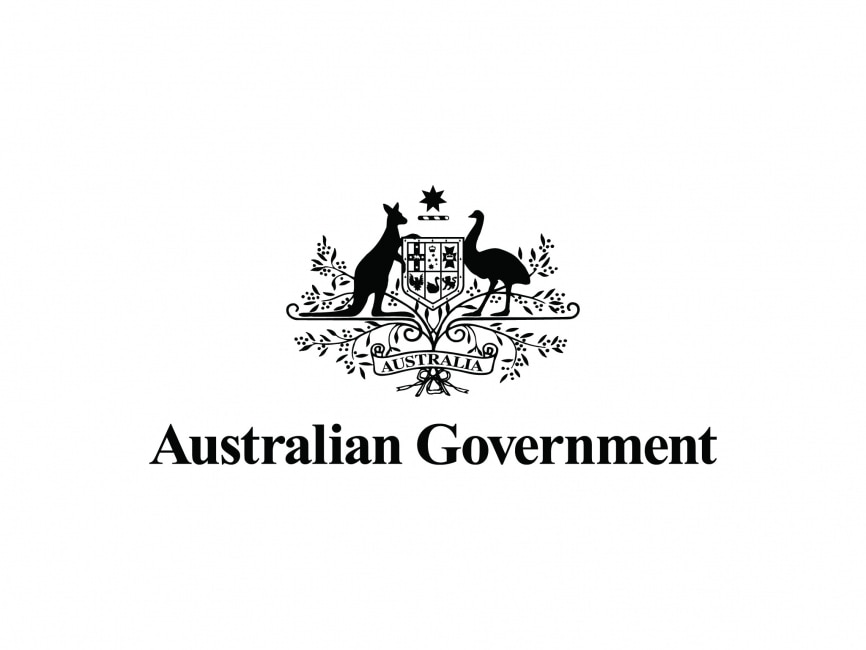
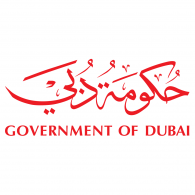
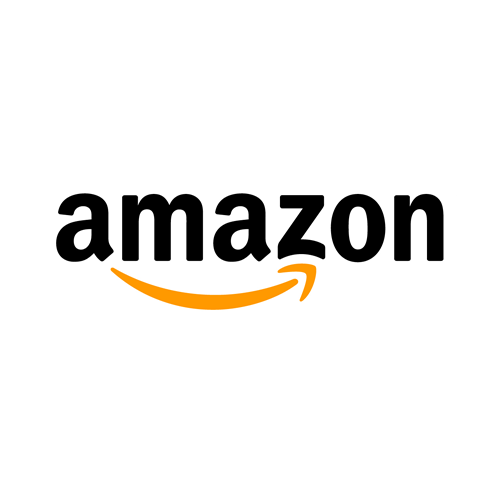
Unveiling the Limitations of AI in Pharmaceutical Research
03 July, 2024
Artificial Intelligence (AI) has been making significant strides in various fields, including pharmaceutical research. However, a recent study led by Prof. Dr. Jürgen Bajorath, a cheminformatics scientist at the University of Bonn, has revealed some limitations of AI in this domain. The team’s research, published in Nature Machine Intelligence, showed that AI programs used in pharmaceutical research largely relied on known data and did not learn specific chemical interactions when predicting drug potency.
Pharmaceutical researchers are constantly on the lookout for efficient active substances to combat diseases. These substances often attach to proteins, typically enzymes or receptors, which trigger a specific chain of physiological actions. In some cases, certain molecules are designed to block undesirable reactions in the body, such as an excessive inflammatory response.
Given the vast number of available chemical compounds, finding the right one can be compared to searching for a needle in a haystack. To expedite this process, scientific models are used to predict which molecules will best attach to the target protein and bind strongly. These potential drug candidates are then scrutinized further in experimental studies.
With the advent of AI, drug discovery research has increasingly started using machine learning applications. One such application is “Graph neural networks” (GNNs), which are designed to predict how strongly a certain molecule binds to a target protein. GNN models are trained with graphs that represent complexes formed between proteins and chemical compounds (ligands).
However, the methodology used by GNNs to arrive at their predictions has been likened to a “black box”, according to Prof. Bajorath. To understand how these AI applications work, the team analyzed six different GNN architectures using their specially developed “EdgeSHAPer” method.
The researchers trained the GNNs with graphs extracted from structures of protein-ligand complexes, for which the mode of action and binding strength of the compounds to their target proteins was already known from experiments. The trained GNNs were then tested on other complexes. The EdgeSHAPer analysis revealed how the GNNs generated seemingly promising predictions.
However, the results were unexpected. Most GNNs only learned a few protein-drug interactions and primarily focused on the ligands. “To predict the binding strength of a molecule to a target protein, the models mainly ‘remembered’ chemically similar molecules that they encountered during training and their binding data, regardless of the target protein,” explained Prof. Bajorath.
These findings suggest that the predictive capabilities of GNNs are largely overrated as equivalent predictions can be made using chemical knowledge and simpler methods. However, the study also revealed that two of the GNN models showed a tendency to learn more interactions when the potency of test compounds increased.
Prof. Bajorath believes that these GNNs could potentially be improved through modified representations and training techniques. However, he cautions that the assumption that physical quantities can be learned based on molecular graphs should be treated with skepticism.
The latest AI news also includes exciting developments in “Explainable AI” at the Lamarr Institute. The team’s approach currently focuses on GNNs and new “chemical language models”. Prof. Bajorath is optimistic about the future of AI in pharmaceutical research, despite its current limitations. He insists, “AI is not black magic,” emphasizing the need for continued research and development of AI tools like the AI images generator and AI text generator to further illuminate the “black box” of AI models.