OUR PARTNERS
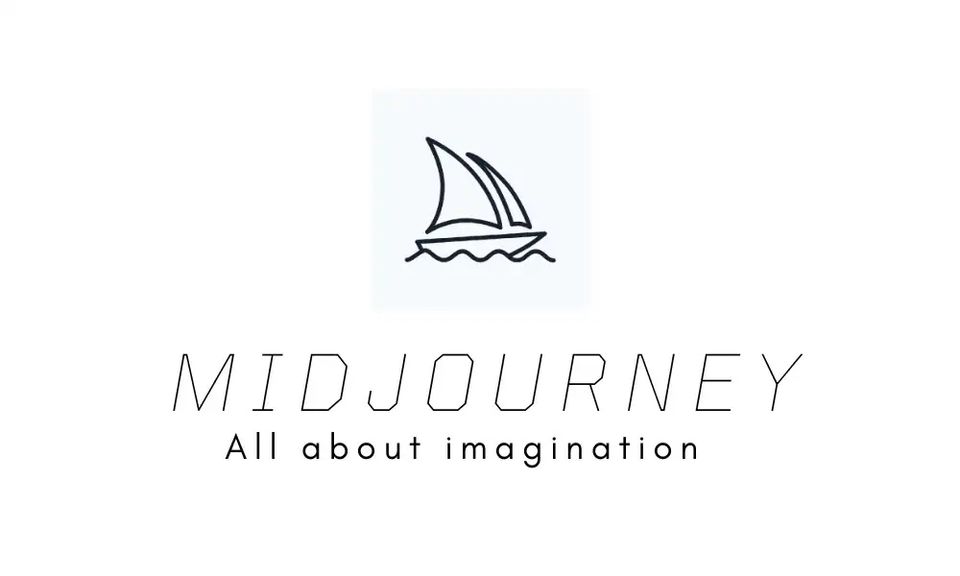
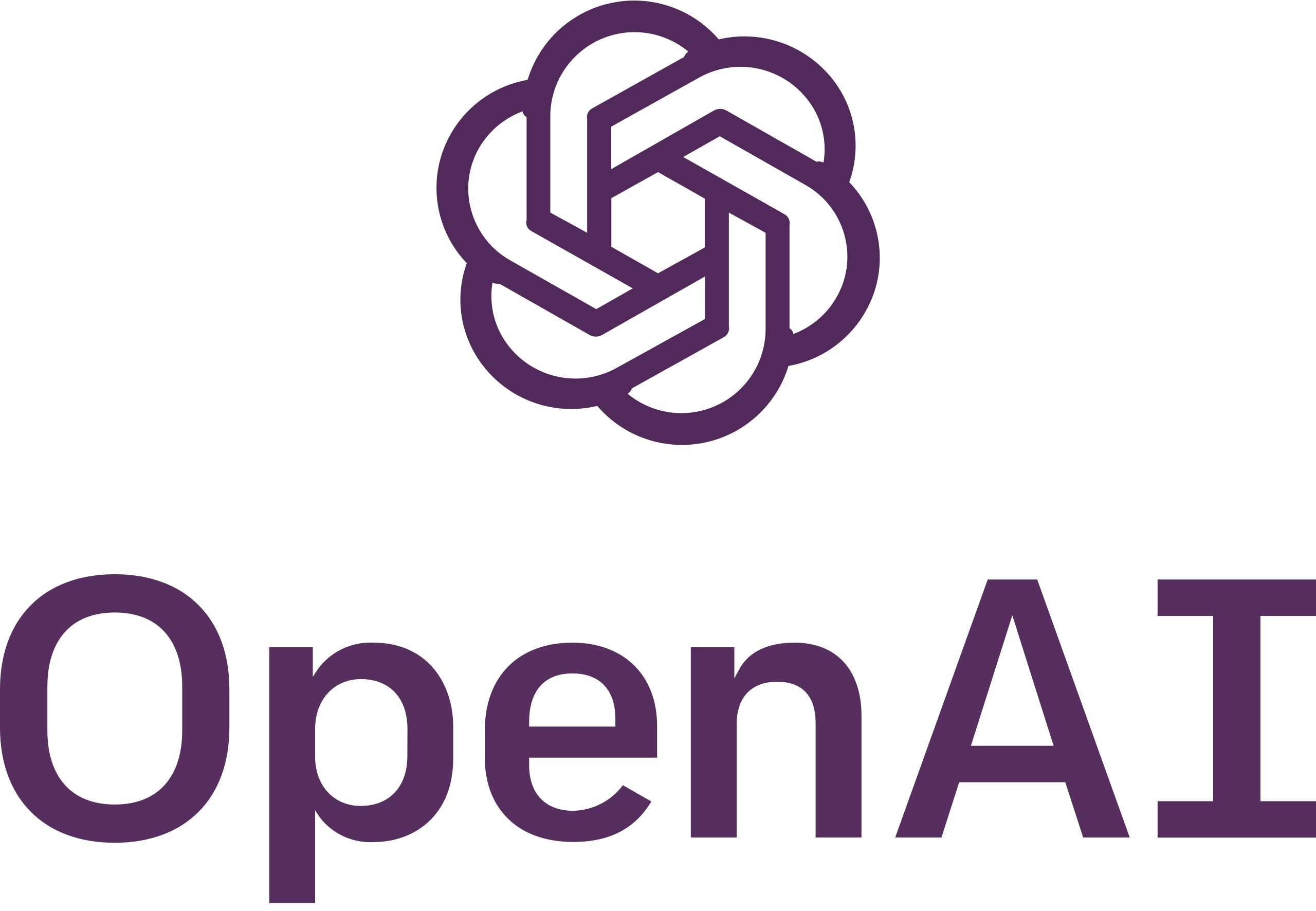
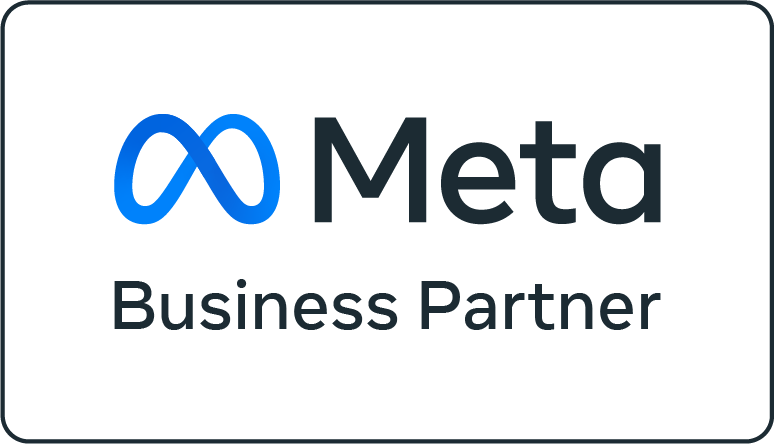
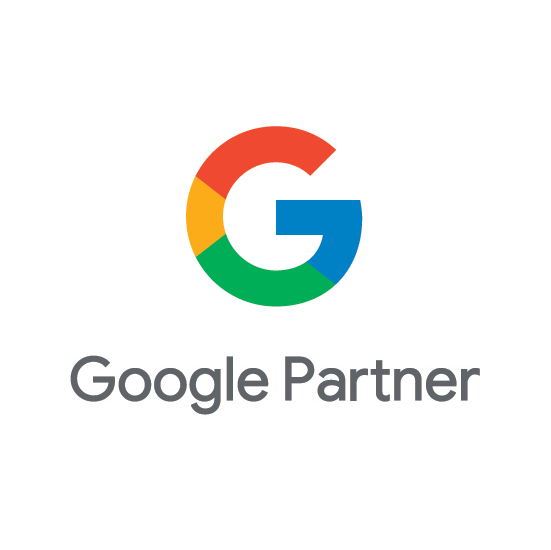
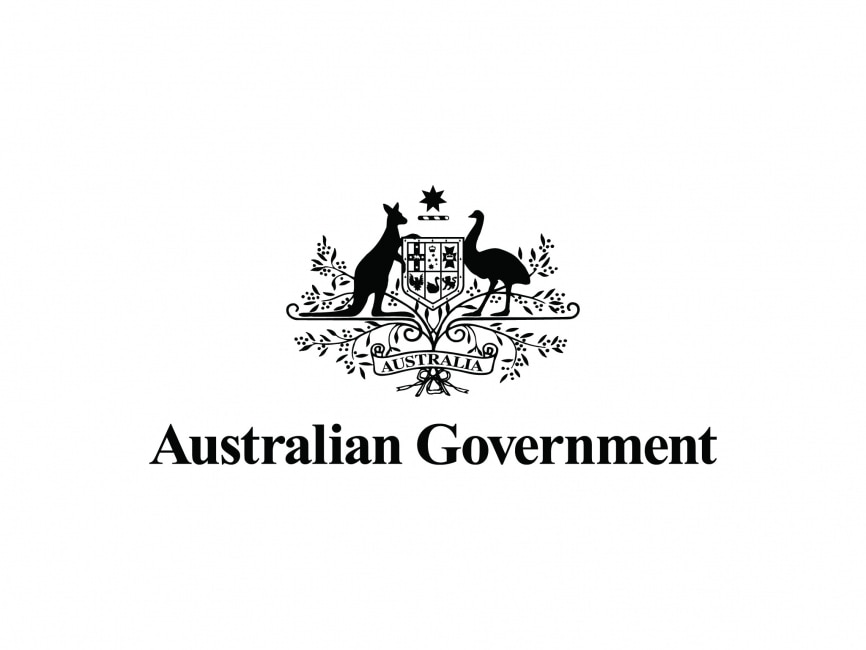
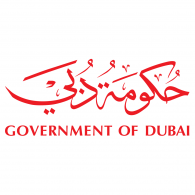
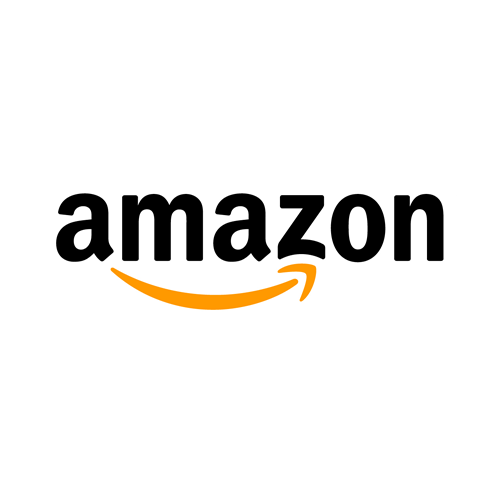
Potential Of On-Device Artificial Intelligence Training Without Cloud Computing
01 July, 2024
Artificial intelligence (AI) has long resided in the vast data centers of tech behemoths, tasked with heavy lifting far beyond the capabilities of our handheld devices. These AI models, pre-trained on gigantic datasets, are then delivered to us through our screens, ready to assist with tasks ranging from generating AI images to predictive text input. However, a groundbreaking shift is on the horizon that holds the potential to decentralize AI training, bringing it directly onto our personal devices.
Imagine a future where your smartphone becomes a hub for AI development, capable of learning and adapting to your unique behaviors and environments in real-time. This is not simply a flight of fancy, but a transformation that might be on the cusp of reality as technology companies strive to move the training of neural networks from the cloud to the device in your hand.
One of the prime advantages of on-device AI training is the immediacy it offers. Without the need to connect to remote servers, AI can learn from your local data on the fly, offering personalized and responsive functionality while ensuring your privacy is guarded by not transmitting sensitive information to external servers.
This leap towards on-device training could dramatically expand what neural networks can do. These intelligent systems could adapt to your routines, recognize the nuances of your day-to-day life, and respond with contextual awareness. From AI video generators capturing and learning from the world as you see it, to ai text generators adapting to your writing style, the possibilities for personalized AI tools are boundless.
Behind the scenes, substantial progress is paving the way for on-device AI training. Apple, for instance, has been exploring how to run more sizable generative neural networks on mobile devices. At the same time, Google has introduced TinyML, an approach tailored for running neural networks on devices with minimal power requirements.
Yet, training these neural networks requires significantly more computational resources than simply running them. To address this, researchers are developing ingenious solutions to minimize the resources needed for on-device training. Selective updating of neural net weights, or transfer learning techniques like MIT’s TinyTL, refine mostly trained neural networks while minimizing resource demands.
Still, we must rise to meet the formidable challenge of training large language models (LLMs) on device. These models brim with billions of parameters, necessitating ingenious strategies to manage memory and processing constraints. Research from STMicroelectronics advocates for streamlining neural networks making them more amenable to on-device training.
Other scientists herald the potential of federated learning, where the load of training is distributed across multiple devices, pooling their collective power. This approach not only lightens the burden on individual devices but also enhances the privacy and security of the data involved.
Beyond technical advancements, these endeavors raise tangible, practical implications for everyday AI usage. On-device training can facilitate more responsive and intelligent cybersecurity measures, support the latest ai news & ai tools, and enable the creation of more dynamic and efficient artificial intelligence generated images.
While the evolution of on-device AI training indeed presents an array of technical challenges—from enhancing network communications to accommodate federated learning to refining the mathematical processes inherent in LLMs—the pursuit is relentless.
The ambition is grand: to transform our personal devices from mere consumers of AI capability into active, learning entities that grow smarter with each interaction. This progression promises to redefine our relationship with technology, delivering a future where AI is not only more personal and responsive but also more integrated into the very fabric of our lives.
In conclusion, the potential for on-device AI training marks a paradigm shift in how we think about and interact with artificial intelligence. It’s an exciting time to keep abreast of the latest ai news & ai tools, and watch as our devices evolve from passive receivers of pre-trained intelligence into dynamic partners, learning alongside us and shaping our digital experiences in unprecedented ways.