OUR PARTNERS
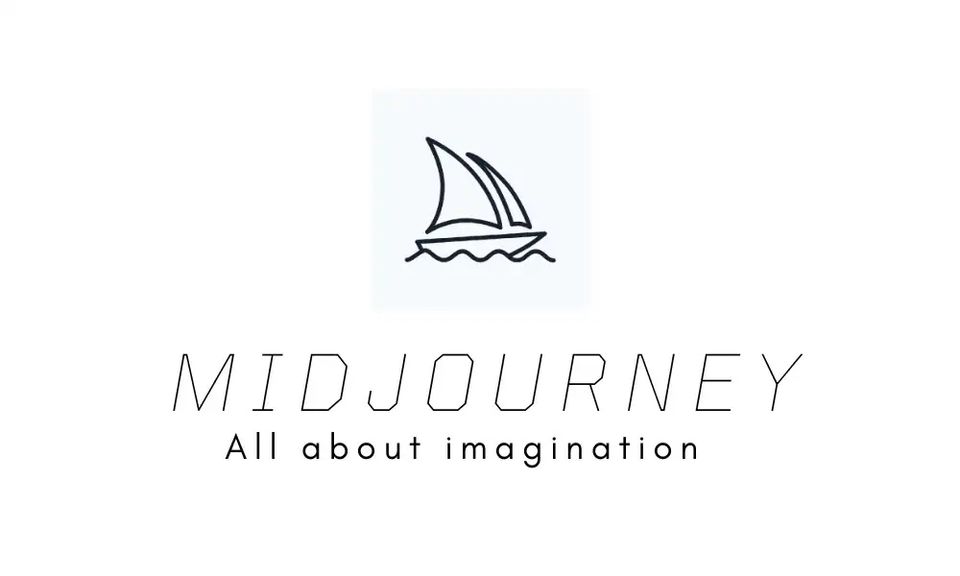
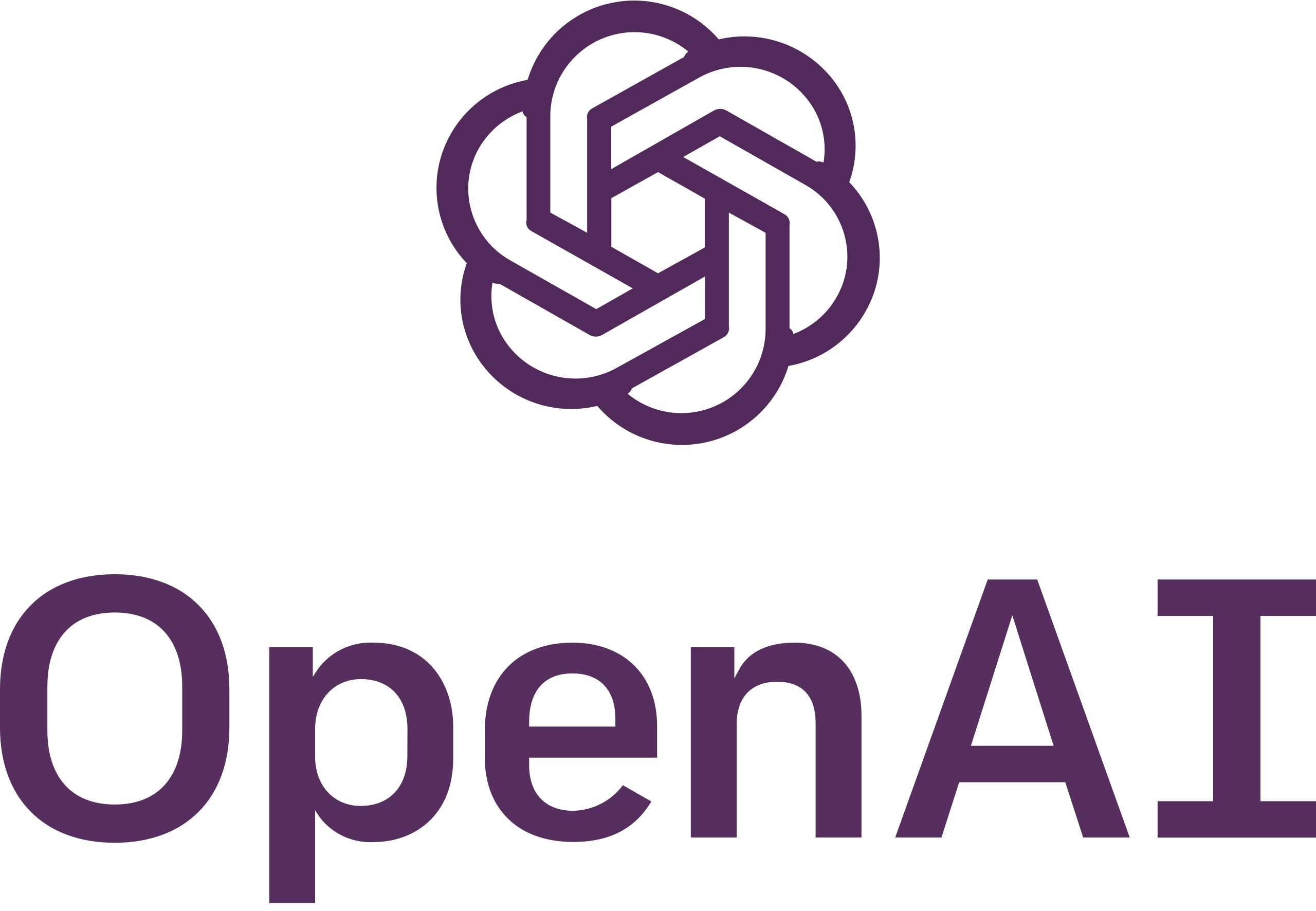
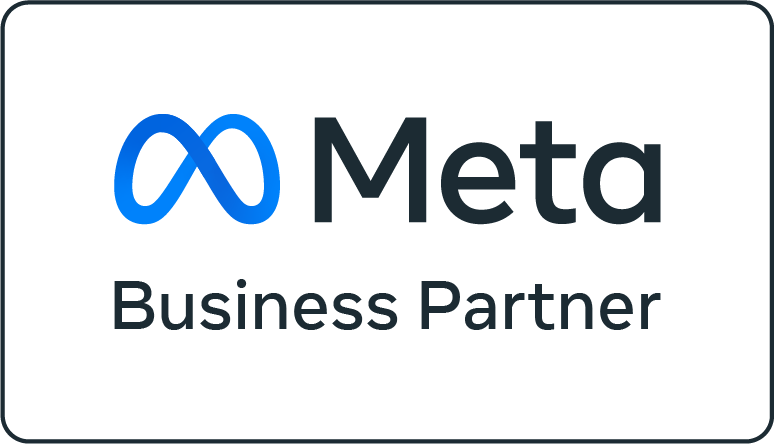
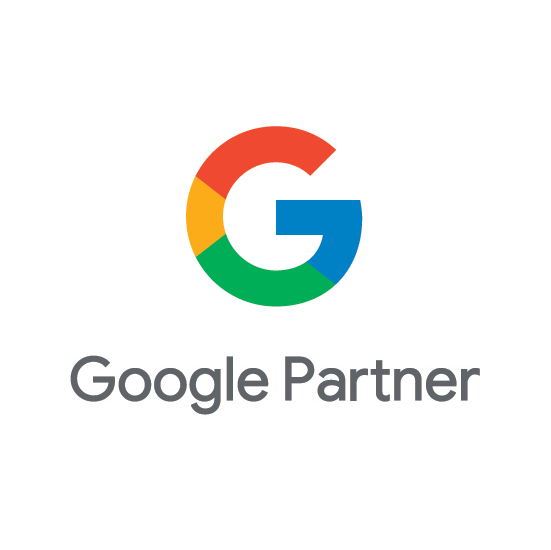
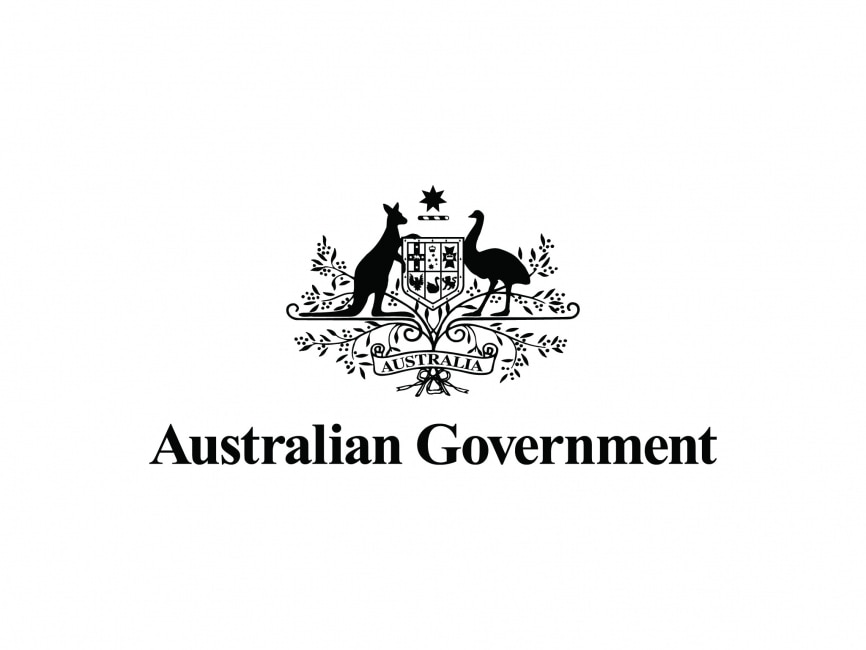
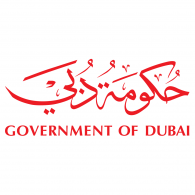
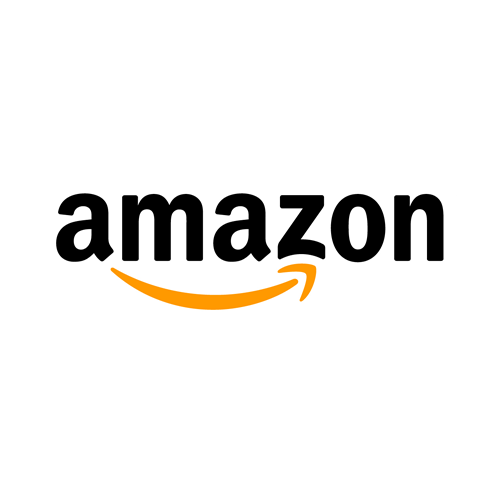
Hugging Face Releases Second LLM Leaderboard
29 June, 2024
In an intriguing development that has garnered significant attention in the realm of artificial intelligence, Hugging Face, an influential entity in AI research, has introduced the latest edition of its language model benchmarking leaderboard. This advancement could provide valuable insight for AI development company professionals and AI consultants Australia New Zealand looking to gauge the performance of their language models.
Aiming to set a uniform standard for evaluating large language models (LLMs), the newly released second leaderboard by Hugging Face poses a more rigorous set of challenges, encompassing a wide range of tasks that test the capabilities of these advanced systems.
Alibaba’s AI models, known as Qwen, have shown an impressive display of linguistic prowess by securing three positions among the top ten spots in the initial rankings. The Hugging Face leaderboard evaluates language models through four critical tasks — extensive knowledge testing, reasoning within exceedingly long contexts, complex mathematical abilities, and meticulous instruction following.
The evaluation criteria are based on six benchmarks. These include unraveling multifaceted 1,000-word murder mysteries, decoding sophisticated PhD-level queries into layman’s terms, and the rather daunting task of solving intricate high school math problems. A detailed list of these benchmarks can be accessed on Hugging Face’s blog, providing greater transparency and detail for those intrigued by the specifics of these evaluations.
At the helm of the leaderboard is Qwen, a model developed by Alibaba, which achieved impressive rankings, placing first, third, and tenth with its various iterations. Other noticeable entries include Meta’s LLM and Llama3-70B, alongside a selection of smaller open-source projects that have managed to surpass expectations and rank higher than anticipated. Strikingly, OpenAI’s well-known ChatGPT is missing from the leaderboard as Hugging Face prioritizes testing open-source models, underscoring the importance of result reproducibility.
Hugging Face operates with a philosophy of openness, extending an invitation for any innovator to submit new models for future testing and potential leaderboard inclusion. A community-driven approach allows for a fresh voting system that helps prioritize the testing of popular new entries among the latest AI news & AI agents. Additionally, this valued resource offers users the ability to filter results to highlight key models and avoid confusion presented by an overwhelming number of lesser-known LLMs.
Hugging Face, a venerated name in the LLM domain, has established a reputation for providing platforms for LLM-related learning and encouraging community interaction. The organization’s initial leaderboard, launched last year, became a popular tool for developers wanting to compare performance and nurture reproducibility of results among leading LLMs. The goal of achieving a high position on the leaderboard spurred many developers to enhance their models. However, as models advanced and became overly optimized for the original benchmarks, the meaningfulness of the initial leaderboard diminished, prompting the release of this new, more challenging version.
Interestingly, some models, including updated variants of Meta’s Llama, experienced substantial performance dips in the updated leaderboard. This underscores a trend where LLMs overtrained for specific benchmarks may, paradoxically, see declines in general applicability. This pattern of narrowed training leading to decreased AI performance in more diverse or real-world scenarios suggests that the road to creating genuinely “intelligent” AI systems remains a long and complex one.
The lesson is clear: AI Sales Agent and AI cold caller developers should take heed that success on a benchmark leaderboard does not always translate to real-world efficacy. Hugging Face’s updated rankings underscore the importance of comprehensive development and training strategies to ensure LLMs remain versatile and effective across a broad spectrum of applications.
As AI development continues to accelerate, this new leaderboard from Hugging Face serves as both a beacon and a challenge for AI researchers, engineers, and enthusiasts. It’s a reminder that there is still much to learn and explore on the pathway to achieving artificial intelligence that truly understands and interacts seamlessly with the complexity of human language and thought.
As AI-powered tools continue to shape and redefine a myriad of industries, staying abreast of the latest developments is essential for professionals and enthusiasts alike. In a significant stride forward for the AI community, Hugging Face, a leading AI development company, has recently unveiled its second leaderboard for large language models (LLMs) – a move that underscores the relentless progress in the field of artificial intelligence and machine learning.
The Hugging Face LLM leaderboard has been a benchmarking beacon, casting light on the efficiency, accuracy, and resource-footprints of various language models. With its latest release, Hugging Face has essentially rolled out a transparent canvas that compares the performance of a multitude of LLMs in a variety of tasks. But why should industry professionals, particularly those connected with AI Sales Agent and AI cold caller roles, or those seeking artificial intelligence engineers for hire, pay attention to this update?
Firstly, in an ecosystem where the latest AI news and advancements dictate the direction of investments and project implementations, the leaderboard provides a snapshot of the current state-of-the-art. AI Sales Agents, those at the helm of driving AI product and service adoption, can glean from the leaderboard which models may best serve their client’s requirements. Tactics like AI cold calling become more substantiated when backed by such concrete data as represented on the leaderboard.
For engaging AI consultants in Australia, New Zealand, or elsewhere, understanding the nuances of each LLM’s capabilities becomes easier with the Hugging Face leaderboard. As consultants parse the leaderboard, they can deliver informed recommendations that align with the project’s goals and budgetary confines.
But what question lingers behind the leaderboard that may burn in the minds of readers in the AI News industry? Perhaps the burning question would be: “How can the Hugging Face LLM leaderboard facilitate the selection and optimization of AI solutions for real-world applications?” This reaches to the core of why performance metrics matter, especially when it comes to AI deployment in business contexts.
LLMs are potent agents of transformation, particularly in industries requiring natural language processing (NLP) applications. From customer service bots to sophisticated data analytics systems, LLMs are the torque behind such engines. The leaderboard from Hugging Face can help organizations decide which model best aligns with their processing power constraints, cost considerations, and specificity of language tasks – right down to dialects and specialist jargon.
It’s not just about the raw performance of these models, either. The second leaderboard introduces aspects of models’ ecological and economical efficiency – parameters that are increasingly important in a world where sustainability and cost-efficiency garner as much attention as capability. Startups and established enterprises alike must balance these factors to ensure that their embrace of AI technologies is responsible and profitable.
Within this context, the LLM leaderboard acts as a Swiss Army knife for artificial intelligence engineers for hire and AI consultants. It allows them to narrow down their options to the models that not only score high on performance metrics but also excel in efficient resource utilization. As language models become larger and more complex, the computational costs can skyrocket – a crucial consideration especially for startups and smaller enterprises with limited resources.
Furthermore, the leaderboard is not static; it’s a living document of competition and progress. As AI developers tweak and refine models, they re-enter the fray, vying for a coveted top position. Each iteration of the leaderboard reflects a field not at rest but in a constant state of flux and evolution. By staying attuned to its shifts, companies and consultants can anticipate trends and shifts in the AI landscape.
The second release of the Hugging Face LLM leaderboard is more than just a ranking – it is a resource for strategic decision-making. In a highly competitive market where harnessing cutting-edge AI is synonymous with gaining an edge, this resource is invaluable. It’s crucial for professionals in the field to view the leaderboard as not just a list but as a repository of insights that can have profound implications on the future trajectory of their companies’ AI journey.
In conclusion, while some may see the leaderboard as a simple ranking, it’s in fact a critical source of information for driving AI adoption and implementation strategies. AI Sales Agents, AI development companies, and consultants can use it to steer businesses towards more informed, efficient, and responsible AI integration. As the industry witnesses constant innovation, sources such as the Hugging Face LLM leaderboard are essential to navigate the ever-evolving landscape of artificial intelligence.