OUR PARTNERS
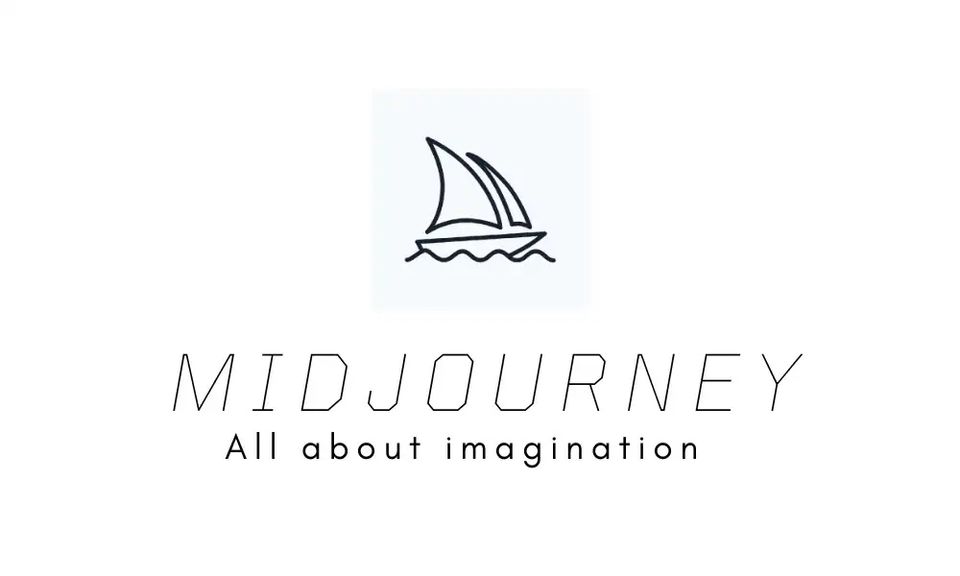
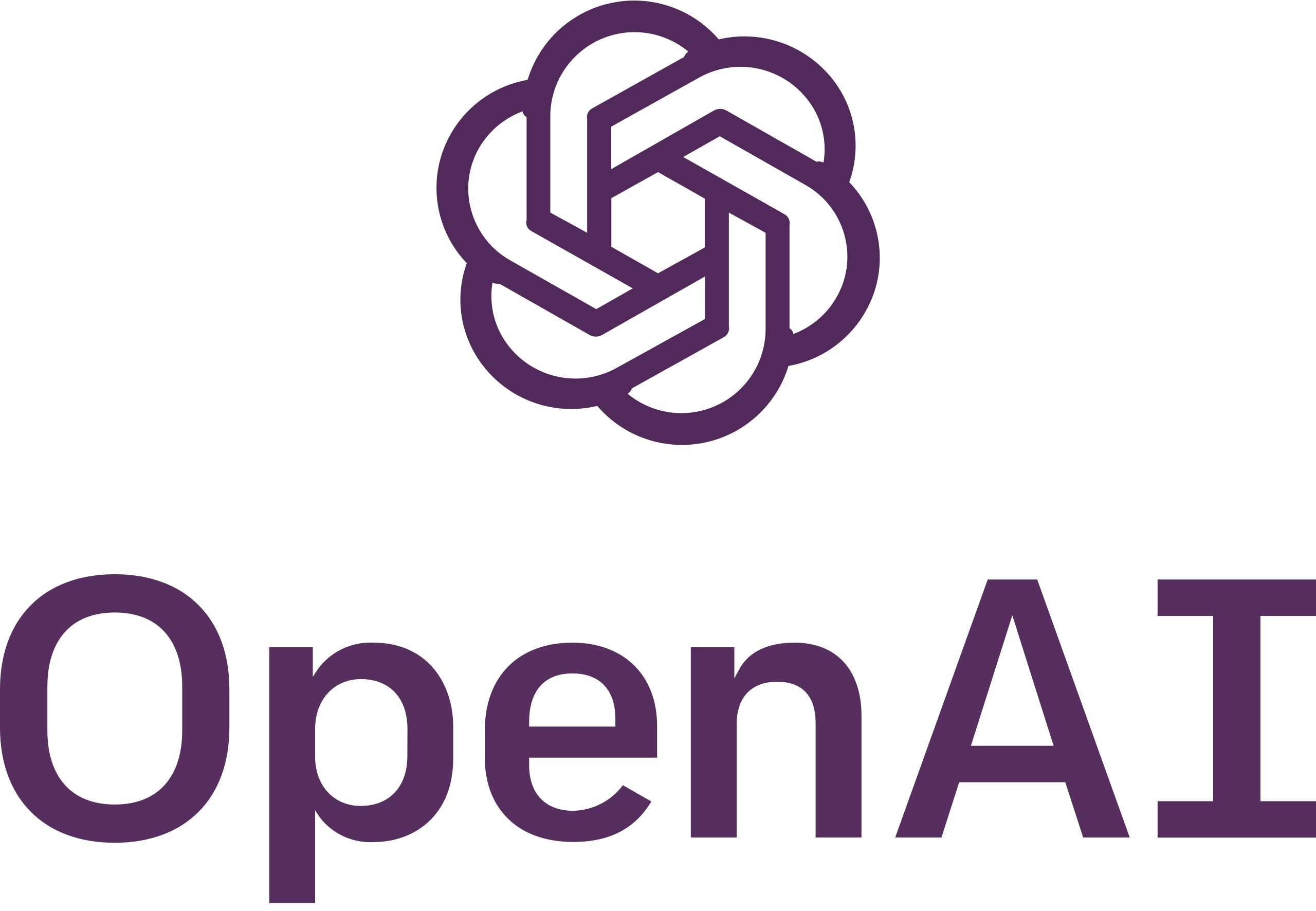
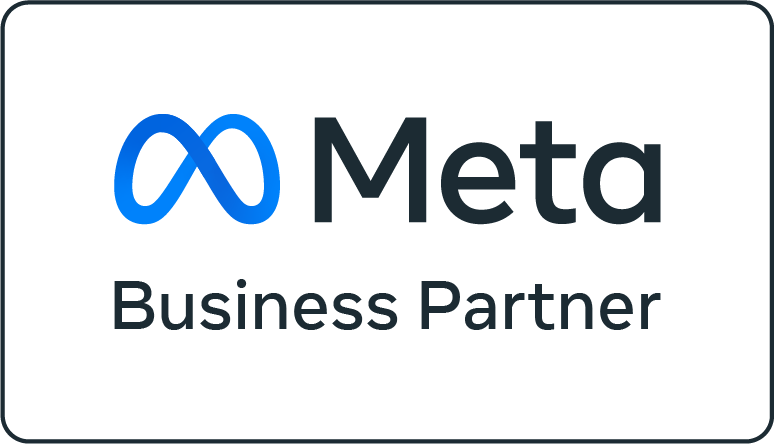
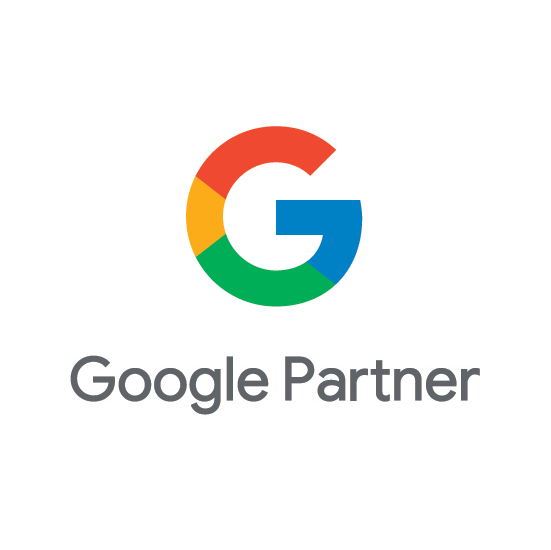
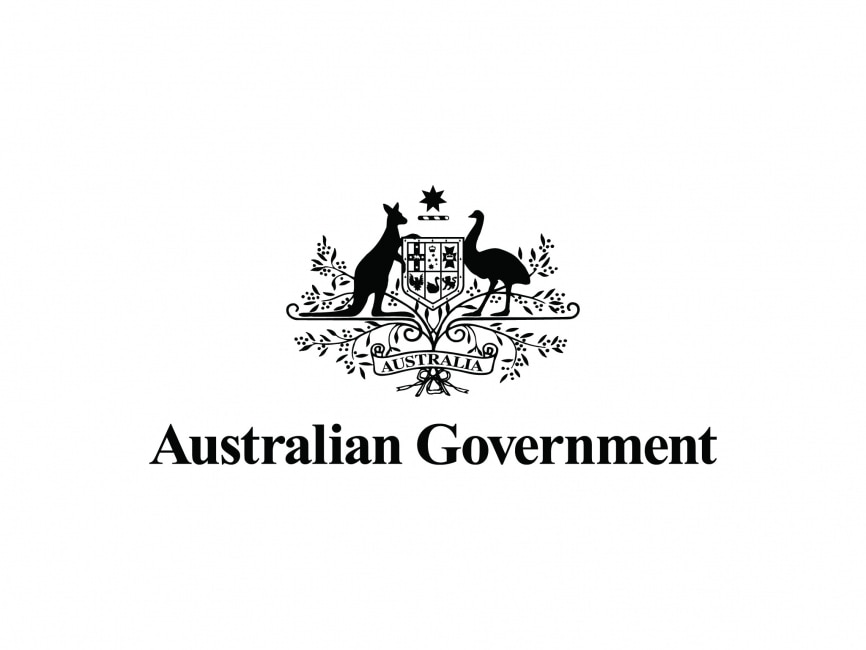
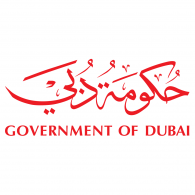
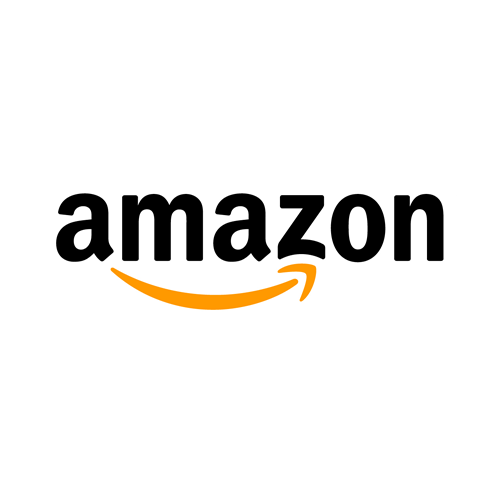
Google’s GraphCast AI Outperforms Traditional Weather Forecasting Models
03 July, 2024
In a groundbreaking development, Google’s artificial intelligence (AI) model, GraphCast, is revolutionizing the field of meteorology by enhancing the precision of weather forecasting. This AI tool has been trained on nearly four decades of historical weather data and is capable of generating a 10-day forecast at six-hour intervals across global locations. This innovative AI model completes the task in less than a minute using a computer no bigger than a small box, a feat that traditionally required an hour or more on a supercomputer the size of a school bus.
GraphCast has proven its mettle by outperforming traditional models, improving accuracy by about 10 percent on over 90 percent of the evaluated weather variables. This innovative AI images generator takes a unique approach to forecasting. It is initially trained to identify patterns in extensive historical weather data and then uses these patterns to generate forecasts based on current conditions. This process is considerably less computationally demanding and can be executed in minutes or even seconds on much smaller computers.
Despite its impressive performance, some researchers have raised concerns about the AI’s ability to accurately predict extreme weather events, primarily due to the rarity of such events in historical data. However, GraphCast has demonstrated its proficiency by reducing cyclone forecast track errors by approximately 10 to 15 miles at a lead time of two to four days, enhancing forecasts of water vapor associated with atmospheric rivers by 10 to 25 percent, and providing more accurate forecasts of extreme heat and cold five to 10 days in advance.
Peter Battaglia, research director at Google DeepMind and co-author of the study, commented on the AI’s performance. He noted that while it might be expected that AI would struggle with rare or unusual weather events, GraphCast performed well in these areas. He suggests that this indicates the model’s ability to capture more fundamental aspects of weather evolution over time, rather than simply identifying superficial patterns in the data.
However, not all experts are convinced. Some argue that while the study supports the idea that AI can make skillful forecasts for most events, it does not dispel doubts about its effectiveness in predicting extreme weather events. They point out that the study provides broad, aggregate statistics of extreme weather forecast skill, but does not detail how the model performs on individual extreme events.
There are other hurdles to overcome before AI models like GraphCast can be consistently used in operational forecasting. Due to limitations in training data and engineering constraints, global AI models are not yet capable of generating forecasts as detailed or as wide-ranging as those from traditional models. This restricts the AI’s usefulness in predicting smaller-scale phenomena, such as thunderstorms and flash flooding, or larger weather systems that can cause significant variations in precipitation over small distances.
Jacob Radford, a data visualization researcher at the Cooperative Institute for Research in the Atmosphere at Colorado State University, highlights another issue. He explains that forecasters play a crucial role in interpreting and communicating information to partners, a task made more difficult by the lack of tools to understand why an AI model makes a particular forecast.
Despite these challenges, the authors of the study maintain that AI models like GraphCast should not be seen as replacements for traditional weather forecasting methods, but rather as tools that can complement and enhance current methodologies. In the latest AI news, these advancements signal a promising future for weather prediction, with AI models like GraphCast leading the way.