OUR PARTNERS
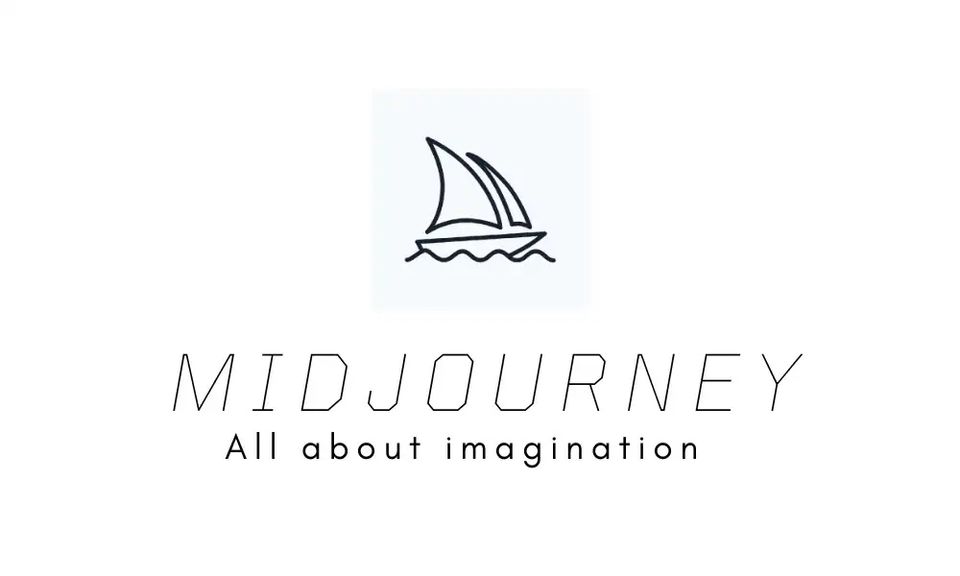
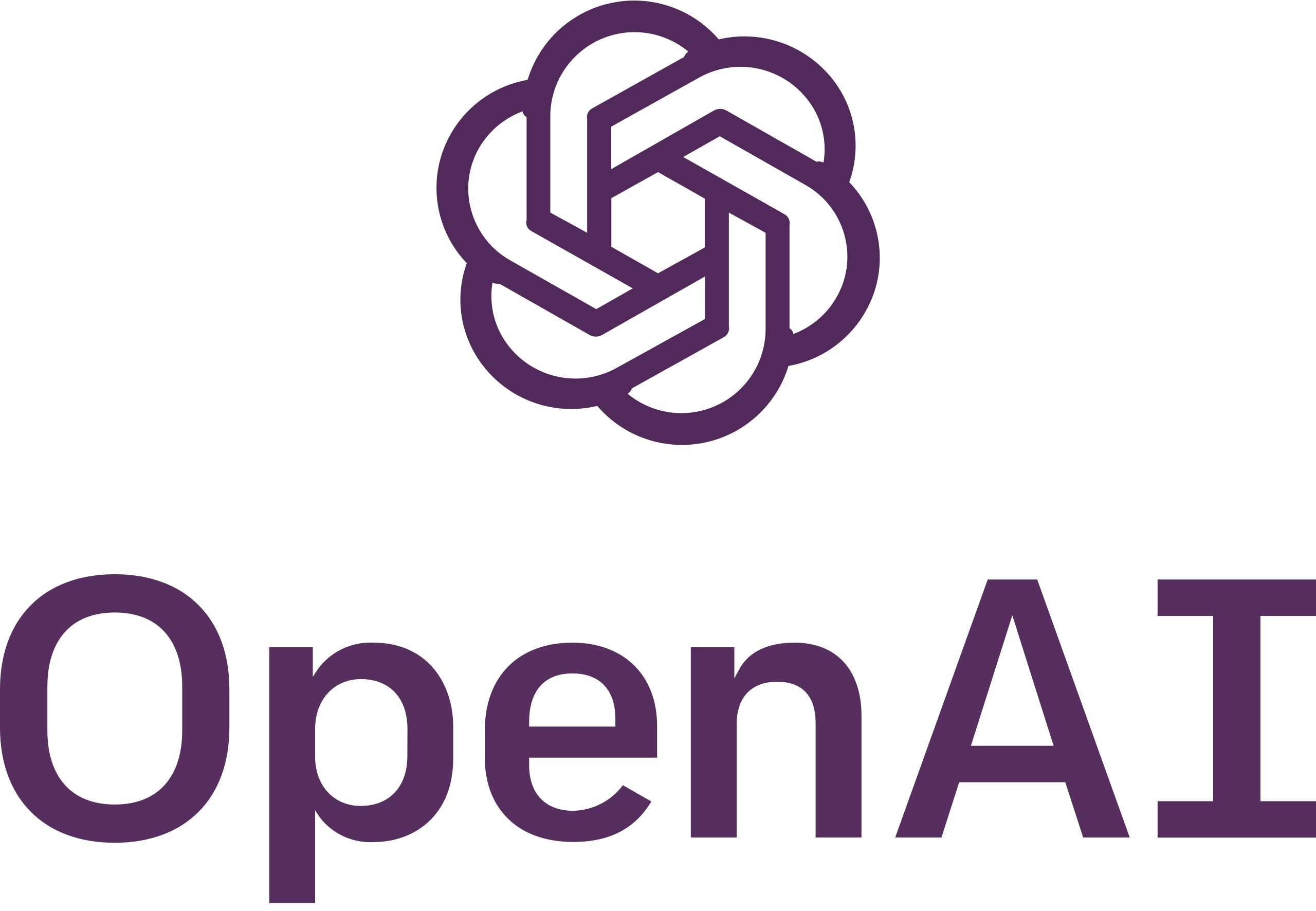
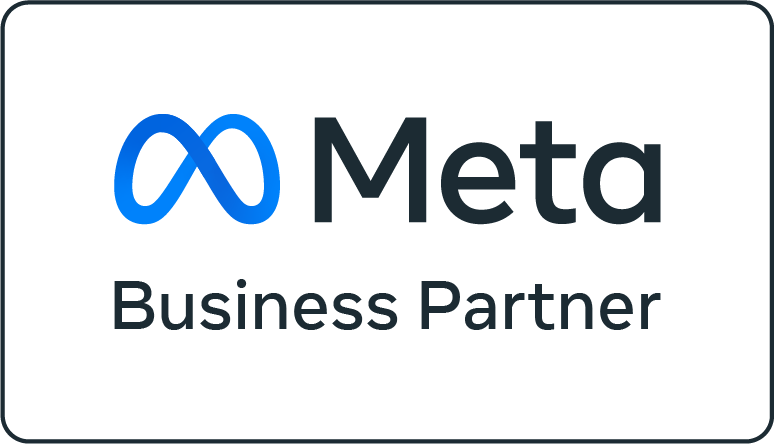
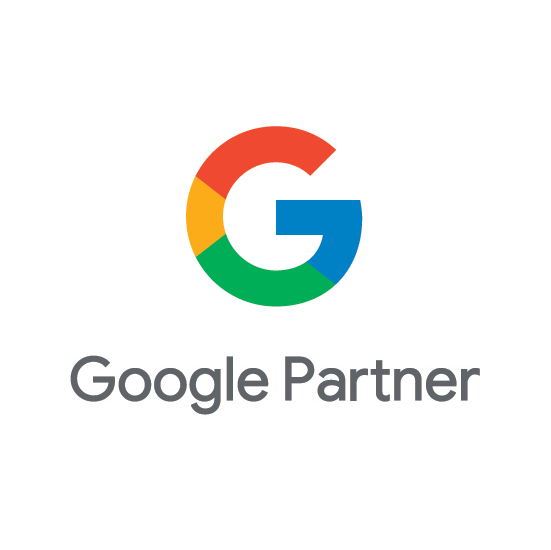
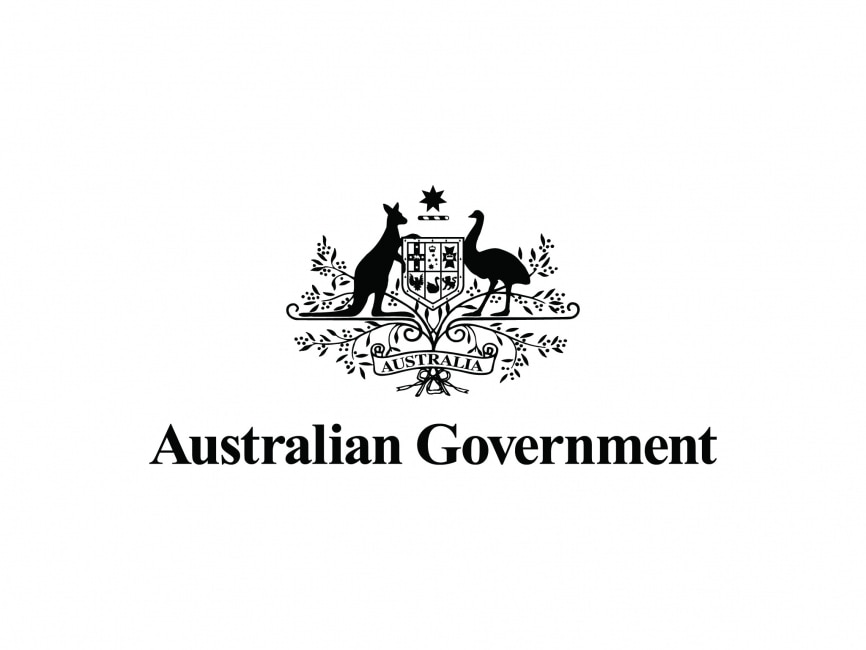
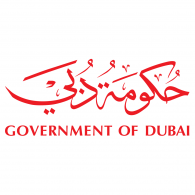
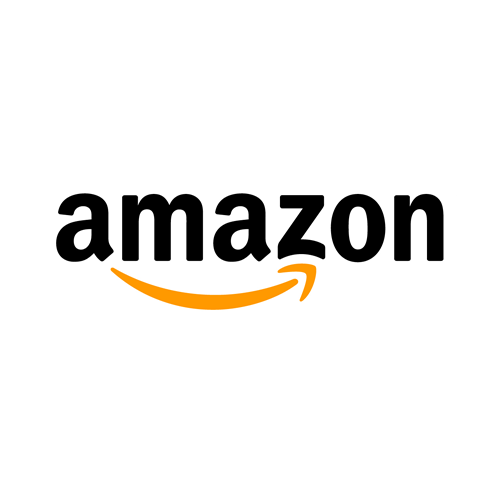
Developed Artificial Intelligence Software Speeds Neural Research Reproducibility
19 June, 2024
In a groundbreaking advancement that promises to revolutionize animal behavior research, a team of scientists from the University of Washington School of Medicine have developed an artificial intelligence software called SimBA (Simple Behavioral Analysis) which simplifies the analysis of intricate animal behaviors. This analysis is critical for understanding the relationship between behavior and brain activity – a connection that is essential to uncovering treatments for human conditions like addiction, anxiety, and depression.
SimBA accelerates this process by using AI to track and categorize behaviors in animals so that these can be accurately associated with specific brain circuits and neuron activities. The latest AI news indicates that such tools are transforming the field, allowing researchers to advance beyond the limitations of traditional manual observation that often struggles to capture the fleeting and nuanced nature of social interactions.
Trained observers would typically watch animals, like mice, and document their responses to stimuli – a method both time-consuming and susceptible to variability. Recognizing components such as posture or quick tail movements presents significant challenges and risks the loss of critical data. AI technology, such as SimBA, overcomes these hurdles with its ability to rapidly record intricate details and more precisely mirror these with simultaneous, rapid neural activity.
Among the most exciting features of SimBA, reported in Nature Neuroscience, is its accessibility. Created by Simon Nilsson and graduate student Nastacia Goodwin under the guidance of assistant professor Sam Golden, SimBA’s open-source platform, equipped with an intuitive graphical interface, demands no specialized computing skills. This innovative tool has seen substantial adoption across the scientific community, across various domains from rodent to zebrafish labs.
Despite the promise and excitement surrounding AI tools in behavioral science, challenges have persisted. Researchers noted that differing methodologies often led to inconsistent results across studies. This variation was partly due to subjective definitions of observed behaviors and the opaque nature of AI systems, commonly referred to as a “black box,” where the decision-making processes are not always clear.
In an insightful attempt to address these issues, the SimBA development team integrated a machine-learning explainability approach that utilizes the Shapely Additive exPlanations (SHAP) score. The SHAP score innovatively assesses the importance of individual features in the AI’s decision-making by determining the impact each has on the algorithm’s predictions.
Through this method, the elusive “black box” of AI decision-making is unraveled, enabling researchers to quantitatively define behaviors sans the subjectivity of individual interpretations. With SHAP scores, scientists can now achieve a deeper understanding of why the AI categorizes behaviors in specific ways, offering a glimpse into the AI’s “thought process.”
AI-generated images and videos have been instrumental in enhancing the understanding of animal behavior, offering valuable visual aids that complement the text-based outputs of AI analysis tools. This harmonized use of an AI images generator and AI video generator underscores the breadth with which AI technologies can enrich scientific research.
By facilitating objective comparisons between different research labs, SimBA fosters a more standardized and reproducible approach to behavioral studies. Previously, inconsistencies in neural data might have been dismissed as resulting from a plethora of confounding variables. However, as Dr. Golden emphasizes, behavioral differences can no longer be overlooked as SimBA enables a direct and clear interpretation.
In essence, SimBA stands as a modern testament to the power of artificial intelligence generated images and algorithms, not just in advancing scientific research but also in promoting a consistent and collaborative approach across the globe. As research continues to evolve with the implementation of AI tools like SimBA, we can expect a surge in cross-lab reproducibility and interpretability—cornerstones for generating robust and transformative scientific insights.