OUR PARTNERS
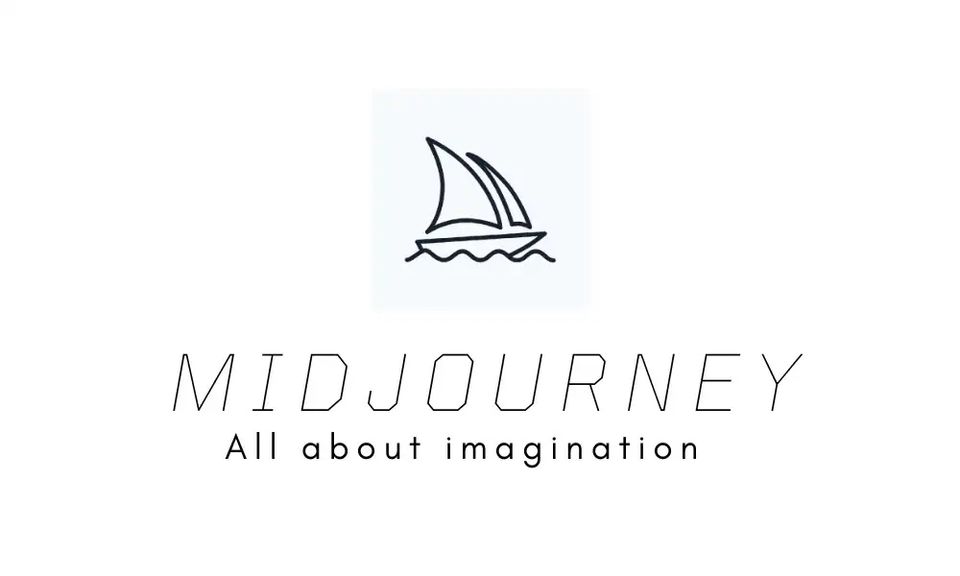
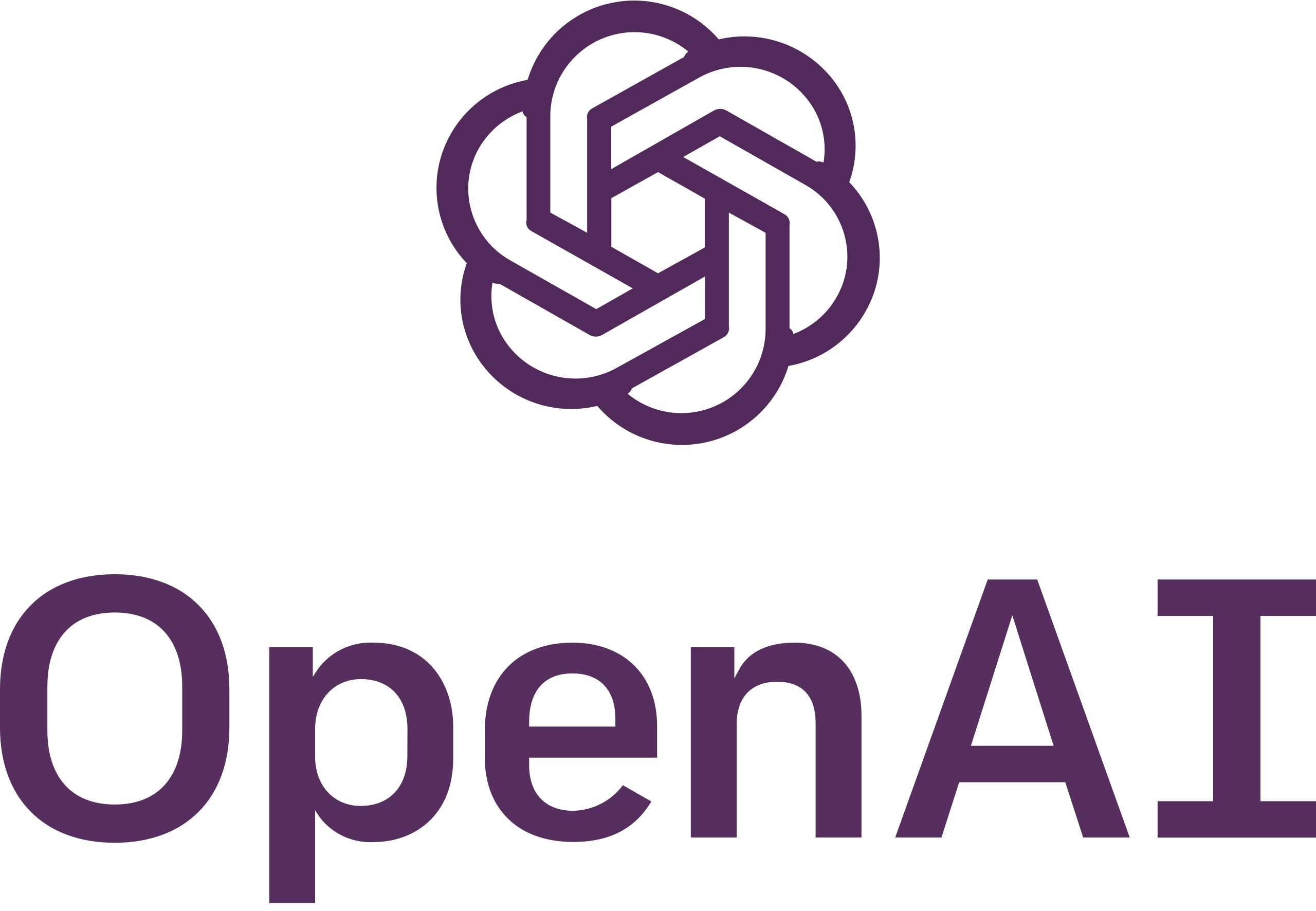
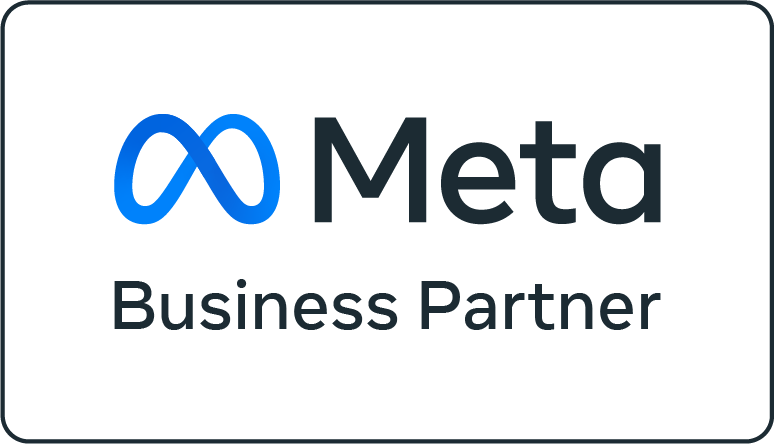
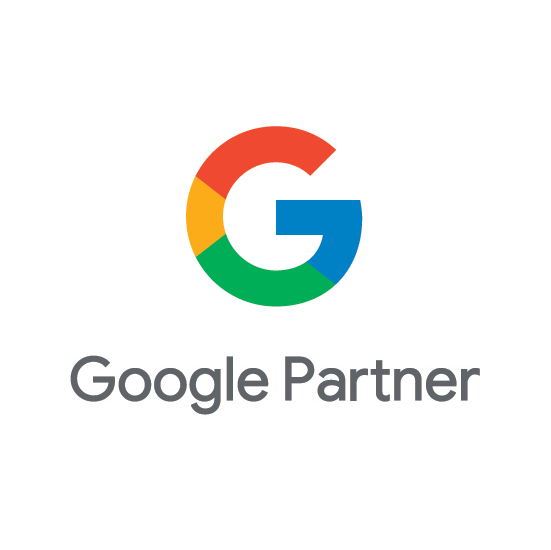
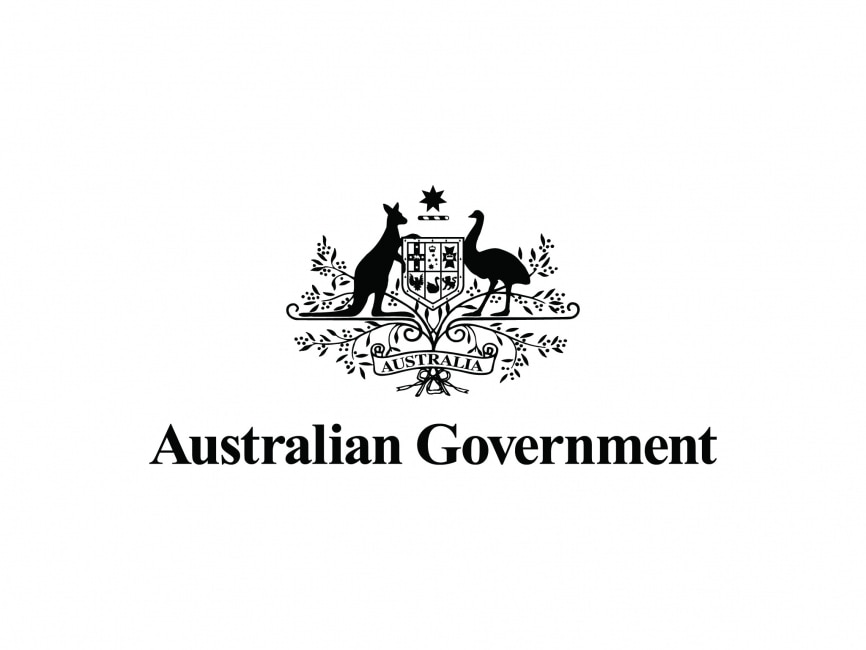
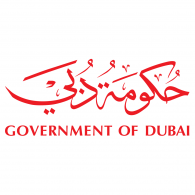
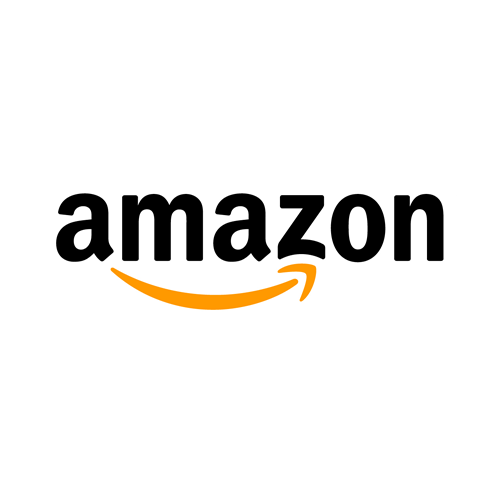
Creating Intelligence: The Complexities of Artificial Intelligence
30 June, 2024
Artificial intelligence, a term that conjures images of sentient robots and self-thinking computers, remains a field in continuous evolution, shaping the very fabric of society and industry as we know it. This transformative technology turns machines into repositories of intelligence, enabling them to engage in problem-solving with little to no human intervention.
Imagine trying to distinguish between images of cats and dogs. For a machine, this isn’t as straightforward as it is for a human eye. Hand over thousands of such images to an AI, and it embarks on the task by working with intricate algorithms and vast stores of data. It could, for instance, analyze various attributes from the images – such as facial structure, eye shape, fur patterns, body size – in a multidimensional graph that facilitates accurate classification.
The notions of “AI images generator” or “AI video generator” stem from such capabilities, where machines use sophisticated software to discern, create, and manipulate visual content. These tools serve not merely for categorization but also for generating new, artificial intelligence generated images that are often indistinguishable from those captured by human hands.
However, complexity escalates with more nuanced tasks, such as instructing a driverless car algorithm to assess potential hazards and act accordingly. Here, the machine must weigh numerous variables and make split-second decisions, with safety implications no less critical than life and death.
A machine’s capacity to learn is categorized broadly into three learning styles: supervised, unsupervised, and reinforcement learning. Supervised learning involves clearly labeled data assisting the AI in making connections and understanding expectations. Unsupervised learning offers no labels, challenging the machine to deduce structures and patterns within the data. Reinforcement learning injects a competitive edge by rewarding the AI for successful outcomes, encouraging adaptation and optimization over time.
Underpinning these learning methodologies is the complex structure known as artificial neural networks (ANNs). ANNs draw inspiration from the human brain, mimicking the intricate web of neurons and synapses. Their nodes act like neurons, while the inter-node connections represent synapses. These networks consist not only of node structures but two key elements: activation functions and weights.
Activation functions decide whether a node should ‘fire’ or activate, analogous to a neuron’s response to stimuli. Weights assign significance to the inputs; these are adjustable factors that ultimately determine how influential a given input is in the network’s decision-making process.
By modifying weights and measuring outputs through activation functions, ANNs learn to optimize responses, much like teaching a child to identify patterns through repeated exercises.
Consider a machine tasked with discerning a pet’s breed from an image. Individual nodes might assess fur color, tail length, or ear shape, each assigning different weights to these features based on their learned importance. The outputs of these nodes feed into other nodes until the system concludes whether the animal in question is, say, a Corgi or a Siamese cat.
Advancements in AI haven’t stopped at mere categorization tools. Generative AI, for example, embarks on a different route altogether. Unlike a traditional classifier concerned with sorting, generative AI models, like the ones used in an ai text generator, create new content based on the patterns they’ve learned. These models, known as Large Language Models (LLMs), are exceptional in their capacity to predict the next word in a sentence, a functionality around which tools like ChatGPT are centered.
Crucially, generative AI does not just fit the words in appropriate sequences but attempts to understand the underlying real-world processes that influence text creation. A feat achieved, for instance, by ChatGPT, leveraging a staggering 100+ billion parameters.
The field of artificial intelligence continues to astonish with its leaps forward. From the latest AI news & AI tools to groundbreaking predictive models, the potential is seemingly limitless. As technology evolves, so too does the intelligence replicated within the machines we increasingly rely on. As we forge ahead, it’s clear that AI will continue to assimilate more complex nuances of human cognition, endeavoring to solve problems once thought the sole domain of biological brains.